In this video I explain how we can use the equation for a normal curve to generate a probability density function and use that to estimate probabilities. While normal curves may differ in where they are centered and how spread out they are, the probabilities associated with comparable sections will always be the same. This means that we can generate a standard version of the normal curve which is centered at 0 with a standard deviation of 1, and then use this estimate the probabilities for any normal curve by converting to standard deviation units, known as Z-scores. The Empirical Rule, or 68-95-99.7 rule tells us the percentage of scores in a normal distribution that will fall within 1, 2, and 3 standard deviations of the mean.
Video Transcript
Hi, I’m Michael Corayer and this is Psych Exam Review. In the previous video I introduced the idea of a probability density function and this is a line or curve that represents the distribution of a variable in a population. And what we saw is that we can use this to estimate probabilities for any given range of values of the variable. So we can select the range of values and if we find the area under the curve this will tell us the percentage of scores in the population within that range of values. And what that means is we can estimate the probability associated with that range of values. So if I look at an area under the curve between, you know, one point for x and another point for x, and I find an area of 0.1 that would mean that 10% of scores in the population fall within that range. And that means if I randomly select a score from the population it would have a 10% chance of falling in that range.
And when we do this we shift from thinking about the details of our sample to estimating for the population because we want to be able to do this for any range of values for X. But of course not all values of X are going to show up in our sample but we still think that they exist in the population. And so what we do is we use an equation in order to draw this probability density function, right? We take the estimates of the parameters which we get from our sample, the population mean mu, and the standard deviation sigma, and then we use those with an equation in order to generate this line that covers all possible values for our variable X.
The most common way that we do this would be to use the equation for a normal distribution. And so here we have the equation or the function for a normal distribution and so what we do is we put in our estimated parameters for the population, mu and sigma, and then we can put in any value for x and this will tell us the height of the probability density function at that point and that means that we can generate this curve across any possible range of value for X.

So all we do is we put them into this equation. So we have 1 over sigma times the square root of 2 pi, times e and this is a constant; Euler’s number, about 2.718 and then we take that to the minus one half times the value for x minus the population mean divided by the standard deviation, squared. And so we put in our values, we pick any possible value for x and this will tell us the height of the line at that point and so that allows us to draw this perfectly smooth curve across the entire range of possible values for X.
Now this version of the equation is what’s called a parameterized version of the equation and that’s because we’re putting in our estimated parameters and what will happen when you put in your value for mu here is that you’ll get a curve that’s centered at mu. Whatever value you put in for your population mean is where the curve will be centered. And whatever value you put in for sigma here will be the standard deviation of that distribution. And so what that means is if we change those, of course, the curve could be centered somewhere else or it could be more or less spread out.
But what’s important to know is that regardless of where it’s centered or how spread out it is, the probabilities are actually going to be the same because the shape of the curve is going to be the same for any normal distribution. All that’s going to change is where it’s centered and how spread out it is but the probabilities that are associated with comparable sections of that curve are going to be the same for any normal curve. And what that means is we don’t need to generate these probabilities, you know, “brand new” each time we have a normal distribution. We actually can already know them if we know them for any normal distribution. Now a simple example of this is to say that for any normal distribution 50% of the curve is going to fall at or above the mean and 50% of the curve is going to fall at or below the mean and that’s true for any normal distribution. It doesn’t matter what you put in for mu or sigma here, that’s going to be the case. And it’s also going to be the case that the same percentage of scores is going to fall between the mean and one standard deviation or from the mean down one standard deviation.
Now what a standard deviation is might vary, you know, for your measurement it might be 10 points or it might be 20 points or 5 points. That doesn’t really matter; that will just change how spread out the curve looks but the percentages and the probabilities are going to be exactly the same for all normal distributions. And what that means is we could generate these probabilities using just one version of the curve and then all we have to do is convert that to our particular measurement. And so this brings us to what’s known as a standard normal curve.
To create a standard normal curve all we have to do is put in a population mean of zero and a standard deviation of one. And so if we put these values into our parameterized version of the normal equation and then we simplify it, we see we get a version that looks like this:

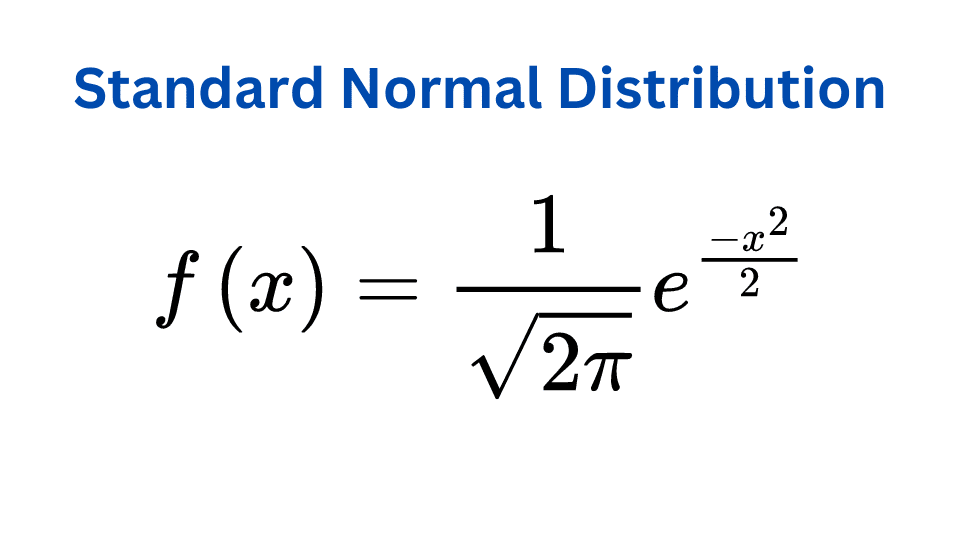
We have 1 over the square root of 2 pi times e to the minus x squared over 2.
Okay so what is this going to do? Well, we put in a population mean of zero and that means we’re going to have the curve centered at 0. And that means that any scores that are above the mean are going to have positive values and any scores that are below the mean are going to have negative values. And by putting in a standard deviation of 1, what we’ve really done is we’ve converted our x axis into being standard deviation units. Because now a value of positive 1 for X means one standard deviation above the mean or a value of minus 0.5 means half a standard deviation below the mean. So what we can do now is calculate all of our probabilities using this version of the curve and it’s going to be very easy to convert those to other measurements.
So here we have a standard normal curve; it’s centered at zero and the x axis here is in standard deviation units; so a score of positive one means one standard deviation above the mean or a score of minus two here is two standard deviations below the mean. And so what we can do is calculate the probabilities that are associated with these standard deviation units and then we could apply those to any data set that’s normally distributed. And this is what’s known as “The Empirical Rule” or you may also see it called the “68-95-99.7 Rule“. And so if we can remember these three numbers then we can very easily estimate some probabilities associated with any normal distribution. So first if we look at the distance from one standard deviation below the mean up to one standard deviation above the mean, so this light blue section here, then the empirical rule says that 68.27% of our scores will fall in this range, or we can just say about 68%. And so what that means is if you have a normally distributed population and you randomly select a score, there’s a 68% chance that score will be within one standard deviation of the mean. And if we go to two standard deviations above and below the mean, so this distance here, then the empirical rule says that we’ll have 95.45% of our scores or we can say about 95% of scores. And if we go to three standard deviations below the mean up to three standard deviations above the mean then we’ll have 99.73% of our scores. So this means that if we have a normally distributed population, almost all of our scores are going to fall within three standard deviations of the mean. And this is also a simple way to test for outliers; we can consider that if you have scores more than three standard deviations from the mean they’re very unlikely to occur, especially if you have a small sample size, and so you might decide that you can identify those as outliers just by using this empirical rule.
So while the empirical rule tells us about these distances in relation to the mean, so we’re thinking about a standard deviation on either side or two standard deviations or three standard deviations, we can also use these numbers, just by remembering these three numbers here 68, 95, and 99.7, we can also estimate other distances in standard deviation units. So if I want to find some other sections I can use the empirical rule to sort of work out these different sections of the curve. So for example if I wanted to know, what’s the probability that I get a score that is greater than one standard deviation above the mean? So from this point and above, what percentage of scores will fall in that range?
Well, I can use the numbers that I memorized from the empirical rule to figure that out rather easily. I can say first of all I know the left half of the curve will be 50% of the curve, so half the curve will be below the mean, and then I just have to think about how large is this section right here? And so if one standard deviation below the mean up to one standard deviation above the mean is 68% of scores then this half must be 34% of scores. And so now I just have to total up how many scores I’ve accounted for so far; I have 50% on this side of the mean then I have another 34% and that means I have 84% of scores and that means there must be 16% of scores remaining above that point. And so the probability of randomly selecting a score and having it be more than one standard deviation above the mean must be 0.16 or 16%.
And of course I could do this for other values as well. I could say, what if I want to know the probability that a score is more than two standard deviations below the mean? So I’m thinking about this point here and I want to know, what’s this small section of the curve on the left here? Well ,again I can use the numbers from the empirical rule. First of all I know I have 50% of scores above the mean and then I know here I have 34% of scores, which we just saw, and then I just have to figure out, well, what is this section right here? I can say well previously we went from 68 to 95 so we increased by 27 and then I’m taking only half of that because that was on either side of the mean, and so that means there must be 13.5% of scores in this section. And so again I can total up what I have above that point; I’d have 50 + 34 + 13.5 and that would tell me that above that point of two standard deviations below the mean must be 97.5% of scores, therefore this remaining section must be about 2.5% of scores. And so if I randomly select a score from the population, the probability that it’s going to be more than two standard deviations below the mean would be 2.5% or 0.025.
Now if we want to do this for our particular measurement we can do this exact same process; all we have to do is change from thinking about a mean of 0 and change from thinking about standard deviation units as neatly being 1, 2, or 3, to whatever they were in our particular measurement. So I say well what would this look like if my mean were centered somewhere else and if one standard deviation were say 10 points on my measurement? I could use this same process in order to estimate probabilities. So let’s say that I’ve collected a sample from a normally distributed population, or at least I believe it’s a normally distributed population, and I’ve estimated the population mean to be 65 and the standard deviation to be 10. So what I can do, because I’m dealing with a normally distributed population here, is I can estimate probabilities using the empirical rule. All I have to do is adjust for the fact that now my curve is not centered at 0, we have a mean of 65, and instead of standard deviation units of 1, 2, or 3, we have a standard deviation of 10. And so all we have to do is convert our scores on this particular measure into standard deviation units and then we can calculate the probabilities.
So let’s say that somebody scores 75 on this assessment. Well I have to say okay this is their raw score, and this is their score in the original units, but I want to convert to standard deviation units. I want to know how many standard deviations is that in relation to the mean. And so I’m going to say well that’s 10 points above the mean and the standard deviation is 10 so that’s one standard deviation above the mean. And so when I’m doing that what I’m doing is finding what we call a z-score. A z-score is a score in standard deviation units and so to find a z-score what we do is we take the raw score which we’ll call X here, we subtract the population mean, and then we divide by the standard deviation. And so what that’s going to do is convert from a raw score into the score in standard deviation units. And so in this case we had 75 minus 65 divided by 10, and that equals 1. So a score of 75 here has a z-score of positive 1, meaning it’s exactly one standard deviation above the mean.
And so since I know the empirical rule, I can immediately estimate the probability of something like, what’s the probability of getting a score 75 or greater on this assessment? Well if the scores are normally distributed then I know it must be 16% of scores that are going to fall above one standard deviation above the mean. So I can immediately estimate that probability by converting 75 into a z-score and then using the probabilities from the empirical rule. And you also notice with the z-score if we had, for example, a negative value, let’s say we had a score of 35. Well this is below the population mean and so we’re going to end up with a negative value we’re going to have 35 minus 65 divided by 10 and we’re going to get minus 3 and so that means a score of 35 on this assessment would be very unlikely to occur it’s three standard deviations below the mean.
And we can also reverse this process. So we can say well if I know the z-score, if I know how many standard deviation units away from the mean, I can convert that into the raw score for my measurement. So for example let’s say I want to know at what point would be two standard deviations below the mean. So I want to find the raw score from the z-score. And we can just rearrange this equation here and say the raw score is the z-score times the standard deviation, so the number of standard deviations times the standard deviation of your measurement, plus whatever the population mean is.
And so if I say okay what’s the raw score that’s two standard deviations below the mean? So that would be a z-score of minus 2 times a standard deviation of 10 plus our population mean of 65 and we’d see that that would be a raw score of 45. And again I could then use that to estimate probabilities. I might say, what’s the probability of getting a score 45 or lower? And just like we saw before we’d say well 2.5% of scores would fall more than two standard deviations below the mean and so that means that on this measurement, since that’s a score of 45, then only 2.5% of people in the population would be expected to score 45 or lower.
Now of course I’ve used simple examples here to introduce this idea of z-scores and raw scores but a z-score could take any value. We could have a score that’s 1.2 standard deviations above the mean or minus 0.46 standard deviations below the mean and in this case the empirical rule isn’t going to be quite as helpful, because we’re going to have some pretty fine gradations there, and we don’t want to memorize all of these probabilities associated with all of the possible z-scores. And so in this case what we do instead is we look to what we call a z table. And what that’s going to do is tell us the probabilities associated with all of these different possible z-scores and that’s what we’re going to look at in the next video. I hope you found this helpful. If so let me know in the comments, like and subscribe, and be sure to check out the hundreds of other psychology tutorials that I have on the channel, and as always, thanks for watching!